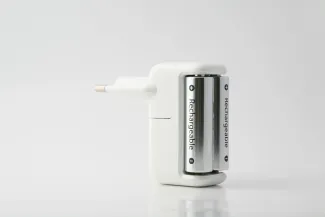
BeCoOptimal
Battery Energy storage system total Cost of Ownership OPTImalisation through physics-informed MAchine Learning
To enhance the performance and reliability of battery energy storage systems, VITO, Octave, and GigaStorage are collaborating on a federal ETF-funded project that integrates machine learning with physicochemical models of battery aging. The project, launched end of 2024, focuses on improving state-of-health estimation, fault diagnostics, and total cost of ownership analysis. This approach supports more accurate monitoring and smarter operational control, enabling battery operators to optimize performance, extend asset life, and increase economic value.
Enhancing Belgium's electricity grid
Battery storage is a key technology for enhancing the flexibility and resilience of the electricity grid in Belgium. By storing excess energy from renewable sources and releasing it when needed, battery storage can help balance supply and demand, reduce congestion and avoid curtailment thereby reducing the need for expensive peaker plants. Moreover, battery storage can provide ancillary services such as frequency and voltage regulation, which are essential for maintaining the stability and equilibrium of the grid.
However, battery storage also faces challenges such as degradation and aging, which affect its performance and lifetime. Yet, battery storage optimisation also needs to consider the impact of its operation on its own lifetime. Therefore, battery storage optimisation needs to balance the trade-off between providing grid services and preserving battery health. Hence, it is important to optimise the operation and management of battery storage systems to maximise their benefits and minimise their costs.
Battery storage lifetime optimisation
The BeCoOptimal project aims to innovate the way battery energy storage systems are currently managed and controlled such that more value can be created in line with the BESS operator’s goals.
The BeCoOptimal approach for battery storage lifetime optimisation is to use a multiobjective optimisation algorithm that considers both grid and battery objectives. The first objective could be to maximise the revenue from providing grid services, while another objective could be to minimise the lifetime degradation cost of the battery.
BeCoOptimal proposes using advanced physics-based algorithms, data analytics and machine learning techniques that can dynamically adjust the charging and discharging patterns of batteries according to the grid conditions, market signals and technical constraints.
VITO's role in BeCoOptimal
VITO, leveraging its extensive expertise and infrastructure, leads the project coordination and is responsible for data analysis, the development of the integrated battery aging model, and the design of the battery control algorithm.
By combining in-house machine learning capabilities with in-house state-of-the-art physicochemical models, VITO focuses on improving state-of-health estimation, fault diagnostics, and total cost of ownership analysis.
These developments lay the groundwork for more intelligent and cost-efficient battery system operation, helping operators make informed decisions that enhance both technical performance and economic return.
Project partners
This project is supported by the Energy Transition Fund.